Identification of a Tumor Microenvironment-relevant Gene set-based Prognostic Signature and Related Therapy Targets in Gastric Cancer
- PMID: 32754268
- PMCID: PMC7392024
- DOI: 10.7150/thno.47938
Identification of a Tumor Microenvironment-relevant Gene set-based Prognostic Signature and Related Therapy Targets in Gastric Cancer
Abstract
Rationale: The prognosis of gastric cancer (GC) patients is poor, and there is limited therapeutic efficacy due to genetic heterogeneity and difficulty in early-stage screening. Here, we developed and validated an individualized gene set-based prognostic signature for gastric cancer (GPSGC) and further explored survival-related regulatory mechanisms as well as therapeutic targets in GC. Methods: By implementing machine learning, a prognostic model was established based on gastric cancer gene expression datasets from 1699 patients from five independent cohorts with reported full clinical annotations. Analysis of the tumor microenvironment, including stromal and immune subcomponents, cell types, panimmune gene sets, and immunomodulatory genes, was carried out in 834 GC patients from three independent cohorts to explore regulatory survival mechanisms and therapeutic targets related to the GPSGC. To prove the stability and reliability of the GPSGC model and therapeutic targets, multiplex fluorescent immunohistochemistry was conducted with tissue microarrays representing 186 GC patients. Based on multivariate Cox analysis, a nomogram that integrated the GPSGC and other clinical risk factors was constructed with two training cohorts and was verified by two validation cohorts. Results: Through machine learning, we obtained an optimal risk assessment model, the GPSGC, which showed higher accuracy in predicting survival than individual prognostic factors. The impact of the GPSGC score on poor survival of GC patients was probably correlated with the remodeling of stromal components in the tumor microenvironment. Specifically, TGFβ and angiogenesis-related gene sets were significantly associated with the GPSGC risk score and poor outcome. Immunomodulatory gene analysis combined with experimental verification further revealed that TGFβ1 and VEGFB may be developed as potential therapeutic targets of GC patients with poor prognosis according to the GPSGC. Furthermore, we developed a nomogram based on the GPSGC and other clinical variables to predict the 3-year and 5-year overall survival for GC patients, which showed improved prognostic accuracy than clinical characteristics only. Conclusion: As a tumor microenvironment-relevant gene set-based prognostic signature, the GPSGC model provides an effective approach to evaluate GC patient survival outcomes and may prolong overall survival by enabling the selection of individualized targeted therapy.
Keywords: gastric cancer; machine learning; prognostic signature; targeted therapy; tumor microenvironment.
© The author(s).
Conflict of interest statement
Competing Interests: The authors have declared that no competing interest exists.
Figures
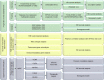
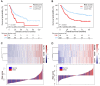
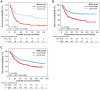
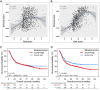
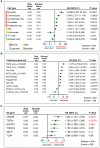
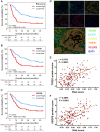
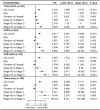
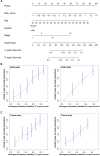
Similar articles
-
Immunogenomic Landscape and Immune-Related Gene-Based Prognostic Signature in Asian Gastric Cancer.Front Oncol. 2021 Nov 5;11:750768. doi: 10.3389/fonc.2021.750768. eCollection 2021. Front Oncol. 2021. PMID: 34804939 Free PMC article.
-
Identification of a novel 10 immune-related genes signature as a prognostic biomarker panel for gastric cancer.Cancer Med. 2021 Sep;10(18):6546-6560. doi: 10.1002/cam4.4180. Epub 2021 Aug 12. Cancer Med. 2021. PMID: 34382341 Free PMC article.
-
Comprehensive Characterization of RNA Processing Factors in Gastric Cancer Identifies a Prognostic Signature for Predicting Clinical Outcomes and Therapeutic Responses.Front Immunol. 2021 Aug 3;12:719628. doi: 10.3389/fimmu.2021.719628. eCollection 2021. Front Immunol. 2021. PMID: 34413861 Free PMC article.
-
Dysregulation of non-coding RNAs in gastric cancer.World J Gastroenterol. 2015 Oct 21;21(39):10956-81. doi: 10.3748/wjg.v21.i39.10956. World J Gastroenterol. 2015. PMID: 26494954 Free PMC article. Review.
-
The role of microRNAs in the gastric cancer tumor microenvironment.Mol Cancer. 2024 Aug 20;23(1):170. doi: 10.1186/s12943-024-02084-x. Mol Cancer. 2024. PMID: 39164671 Free PMC article. Review.
Cited by
-
Use of machine learning-based integration to develop an immune-related signature for improving prognosis in patients with gastric cancer.Sci Rep. 2023 Apr 29;13(1):7019. doi: 10.1038/s41598-023-34291-9. Sci Rep. 2023. PMID: 37120631 Free PMC article. Clinical Trial.
-
A three-gene signature based on tumour microenvironment predicts overall survival of osteosarcoma in adolescents and young adults.Aging (Albany NY). 2020 Dec 3;13(1):619-645. doi: 10.18632/aging.202170. Epub 2020 Dec 3. Aging (Albany NY). 2020. PMID: 33281116 Free PMC article.
-
A Novel Exosome-Relevant Molecular Classification Uncovers Distinct Immune Escape Mechanisms and Genomic Alterations in Gastric Cancer.Front Pharmacol. 2022 Jun 3;13:884090. doi: 10.3389/fphar.2022.884090. eCollection 2022. Front Pharmacol. 2022. PMID: 35721114 Free PMC article.
-
A nomogram model based on the number of examined lymph nodes-related signature to predict prognosis and guide clinical therapy in gastric cancer.Front Immunol. 2022 Nov 2;13:947802. doi: 10.3389/fimmu.2022.947802. eCollection 2022. Front Immunol. 2022. PMID: 36405735 Free PMC article.
-
An integrated analysis of prognostic mRNA signature in early- and progressive-stage gastric adenocarcinoma.Front Mol Biosci. 2023 Jan 4;9:1022056. doi: 10.3389/fmolb.2022.1022056. eCollection 2022. Front Mol Biosci. 2023. PMID: 36660425 Free PMC article.
References
-
- Bray F, Ferlay J, Soerjomataram I, Siegel RL, Torre LA, Jemal A. Global cancer statistics 2018: GLOBOCAN estimates of incidence and mortality worldwide for 36 cancers in 185 countries. CA Cancer J Clin. 2018;68:394–424. - PubMed
-
- Mattiuzzi C, Lippi G. Cancer statistics: a comparison between World Health Organization (WHO) and Global Burden of Disease (GBD) Eur J Public Health. 2019. - PubMed
-
- Chen W, Zheng R, Baade PD, Zhang S, Zeng H, Bray F. et al. Cancer statistics in China, 2015. CA: a cancer journal for clinicians. 2016;66:115–32. - PubMed
-
- Van Cutsem E, Sagaert X, Topal B, Haustermans K, Prenen H. Gastric cancer. Lancet. 2016;388:2654–64. - PubMed
Publication types
MeSH terms
Substances
LinkOut - more resources
Full Text Sources
Medical
Molecular Biology Databases
Research Materials
Miscellaneous